A litany for survival analysis sets the stage for this enthralling narrative, offering readers a glimpse into a story that is rich in detail and brimming with originality from the outset.
Survival analysis is a statistical technique used to analyze the time until an event of interest occurs. It is widely used in fields such as medicine, engineering, and finance to understand the factors that influence the occurrence of events and to make predictions about future outcomes.
Survival Analysis Methods
Survival analysis, also known as time-to-event analysis, is a statistical technique used to analyze the time until an event of interest occurs. In survival analysis, the event of interest is typically a negative outcome, such as death, disease progression, or equipment failure.
There are a variety of survival analysis methods available, each with its own advantages and disadvantages. The most commonly used methods include:
- Kaplan-Meier method
- Cox proportional hazards model
- Accelerated failure time model
The Kaplan-Meier method is a non-parametric method that estimates the survival function from a sample of data. The Kaplan-Meier method is simple to use and interpret, but it can be biased if the data are censored.
The Cox proportional hazards model is a semi-parametric method that models the hazard function as a function of covariates. The Cox proportional hazards model is more flexible than the Kaplan-Meier method, but it can be more difficult to interpret.
The accelerated failure time model is a parametric method that models the survival time as a function of covariates. The accelerated failure time model is more flexible than the Kaplan-Meier method and the Cox proportional hazards model, but it can be more difficult to fit.
The choice of survival analysis method depends on the specific research question and the data available. It is important to consider the advantages and disadvantages of each method before selecting a method.
Assumptions and Limitations of Survival Analysis Techniques
Survival analysis techniques are based on a number of assumptions. These assumptions include:
- The data are independent and identically distributed.
- The censoring mechanism is independent of the survival time.
- The hazard function is constant over time.
These assumptions are often not met in practice. However, if the assumptions are not met, the results of the survival analysis may be biased.
In addition to the assumptions, survival analysis techniques have a number of limitations. These limitations include:
- Survival analysis techniques can be difficult to interpret.
- Survival analysis techniques can be computationally intensive.
- Survival analysis techniques can be sensitive to the choice of model.
Despite these limitations, survival analysis techniques are a powerful tool for analyzing time-to-event data. Survival analysis techniques can be used to identify risk factors for negative outcomes, to compare the effectiveness of different treatments, and to predict the survival time of individuals.
Data Preparation for Survival Analysis
Data preparation is a crucial step in survival analysis to ensure the accuracy and reliability of the results. It involves cleaning, transforming, and selecting data to make it suitable for analysis.
Handling Missing Data
Missing data can occur due to various reasons, such as patient dropout or incomplete records. It is important to identify the pattern of missing data and handle it appropriately. Common techniques include imputation, exclusion, or multiple imputation.
Dealing with Outliers
Outliers are extreme values that can significantly affect the results of survival analysis. They can be caused by measurement errors, data entry mistakes, or genuine extreme events. Outliers should be carefully examined to determine their validity and whether they should be removed or transformed.
Censoring
Censoring occurs when the event of interest has not occurred by the end of the study or when the patient is lost to follow-up. Censoring can be right-censored (event has not occurred), left-censored (event occurred before the study started), or interval-censored (event occurred within a time interval).
Proper handling of censored data is essential for accurate survival analysis.
Data Transformation and Variable Selection
Data transformation can improve the linearity of relationships and stabilize the variance. Common transformations include logarithmic, square root, or Box-Cox transformations. Variable selection is crucial to identify the most important predictors of survival and avoid overfitting. Techniques such as backward elimination, forward selection, or stepwise selection can be used for variable selection.
Model Selection and Evaluation
Selecting the most appropriate survival analysis model for a given dataset is crucial for obtaining reliable and meaningful results. This section provides guidance on model selection and evaluation techniques to ensure the best model is chosen for the task at hand.
Model performance is typically evaluated based on criteria such as concordance index (C-index), Akaike information criterion (AIC), and Bayesian information criterion (BIC). These measures assess the model’s ability to correctly predict the survival outcomes of individuals.
Cross-Validation
Cross-validation is a technique used to evaluate the performance of a model on unseen data. It involves dividing the dataset into multiple subsets, training the model on each subset while evaluating it on the remaining data. The process is repeated for each subset, and the average performance across all iterations provides a more robust estimate of the model’s generalizability.
Bootstrapping
Bootstrapping is another resampling technique that can be used for model selection. It involves randomly sampling with replacement from the original dataset to create multiple new datasets. Each new dataset is used to train a model, and the performance of these models is evaluated.
The model with the best average performance across all bootstrapped datasets is considered the most robust and reliable.
Survival Analysis in Practice
Survival analysis has found widespread applications in various fields, providing valuable insights into time-to-event data. Let’s explore a few examples:
Medicine
Survival analysis plays a crucial role in medical research, particularly in oncology and epidemiology. It helps researchers analyze patient survival data, identify risk factors, and evaluate the effectiveness of treatments. For instance, a survival analysis study can determine the median survival time of patients with a particular type of cancer or assess the impact of a new drug on patient survival rates.
Engineering
In engineering, survival analysis is used to estimate the reliability and durability of products and systems. It helps engineers predict the likelihood of failures or breakdowns over time. For example, a manufacturer might use survival analysis to determine the expected lifespan of a new electronic device or the failure rate of a bridge under different load conditions.
Finance
Survival analysis finds applications in finance to analyze the default rates of loans and bonds. It helps financial institutions assess the risk of default and make informed lending decisions. For instance, a bank might use survival analysis to estimate the probability of a borrower defaulting on a mortgage or the likelihood of a company going bankrupt.
Interpreting Survival Analysis Results
Interpreting survival analysis results involves understanding the key measures and statistics. These include:*
-*Survival curve
A graphical representation of the probability of survival over time.
-
-*Median survival time
The time at which 50% of the subjects have experienced the event of interest.
-*Hazard ratio
A litany for survival analysis can help us understand the factors that contribute to survival. Just as words with the root word hydr are related to water, a litany for survival analysis can help us identify the “water” we need to survive.
A measure of the relative risk of experiencing the event of interest, comparing two groups or conditions.
By analyzing these measures, researchers can draw meaningful conclusions about the factors influencing survival and make informed decisions.
Challenges and Opportunities
Applying survival analysis in real-world scenarios presents both challenges and opportunities:Challenges:*
-*Censoring
Incomplete data due to subjects dropping out or being lost to follow-up can bias results.
-
-*Heterogeneity
Differences in patient characteristics and treatment protocols can make it difficult to generalize findings.
-*Time-dependent covariates
Factors that change over time can introduce complexity into the analysis.
Opportunities:*
-*Advancements in computing power
Computational advancements have made it possible to handle large and complex survival analysis datasets.
-
-*Statistical methods
New statistical methods have been developed to address challenges such as censoring and heterogeneity.
-*Collaboration
Interdisciplinary collaboration between statisticians, clinicians, and researchers can enhance the quality and impact of survival analysis studies.
Advanced Topics in Survival Analysis: A Litany For Survival Analysis
Survival analysis offers advanced techniques to delve deeper into complex survival data. These methods enhance our understanding of survival patterns and provide more comprehensive insights.
Competing Risks
In survival analysis, we often encounter situations where individuals may experience multiple events or outcomes. Competing risks models account for the possibility of competing events, ensuring accurate estimation of survival probabilities.
Time-Dependent Covariates
Time-dependent covariates allow the effects of variables to vary over time. This is crucial when analyzing data where covariate values change dynamically, providing a more nuanced understanding of their impact on survival.
Frailty Models, A litany for survival analysis
Frailty models incorporate unobserved heterogeneity among individuals into the survival analysis. This accounts for the fact that individuals may have different susceptibilities to the event of interest, leading to more precise survival estimates.
User Queries
What is survival analysis?
Survival analysis is a statistical technique used to analyze the time until an event of interest occurs.
What are the different types of survival analysis methods?
There are a variety of survival analysis methods, including the Kaplan-Meier method, the Cox proportional hazards model, and the accelerated failure time model.
How is survival analysis used in practice?
Survival analysis is used in a variety of fields, including medicine, engineering, and finance, to understand the factors that influence the occurrence of events and to make predictions about future outcomes.
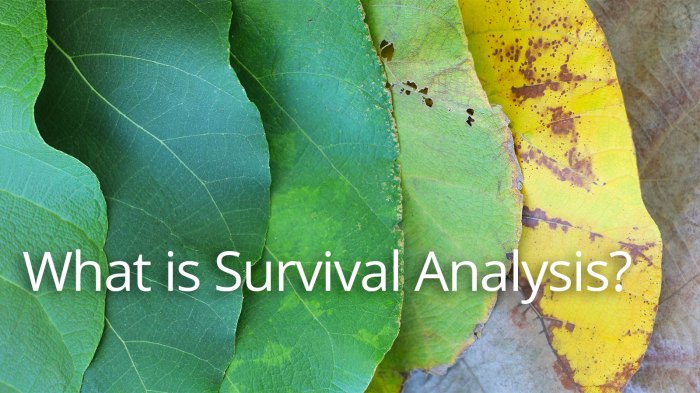